ggml.ai
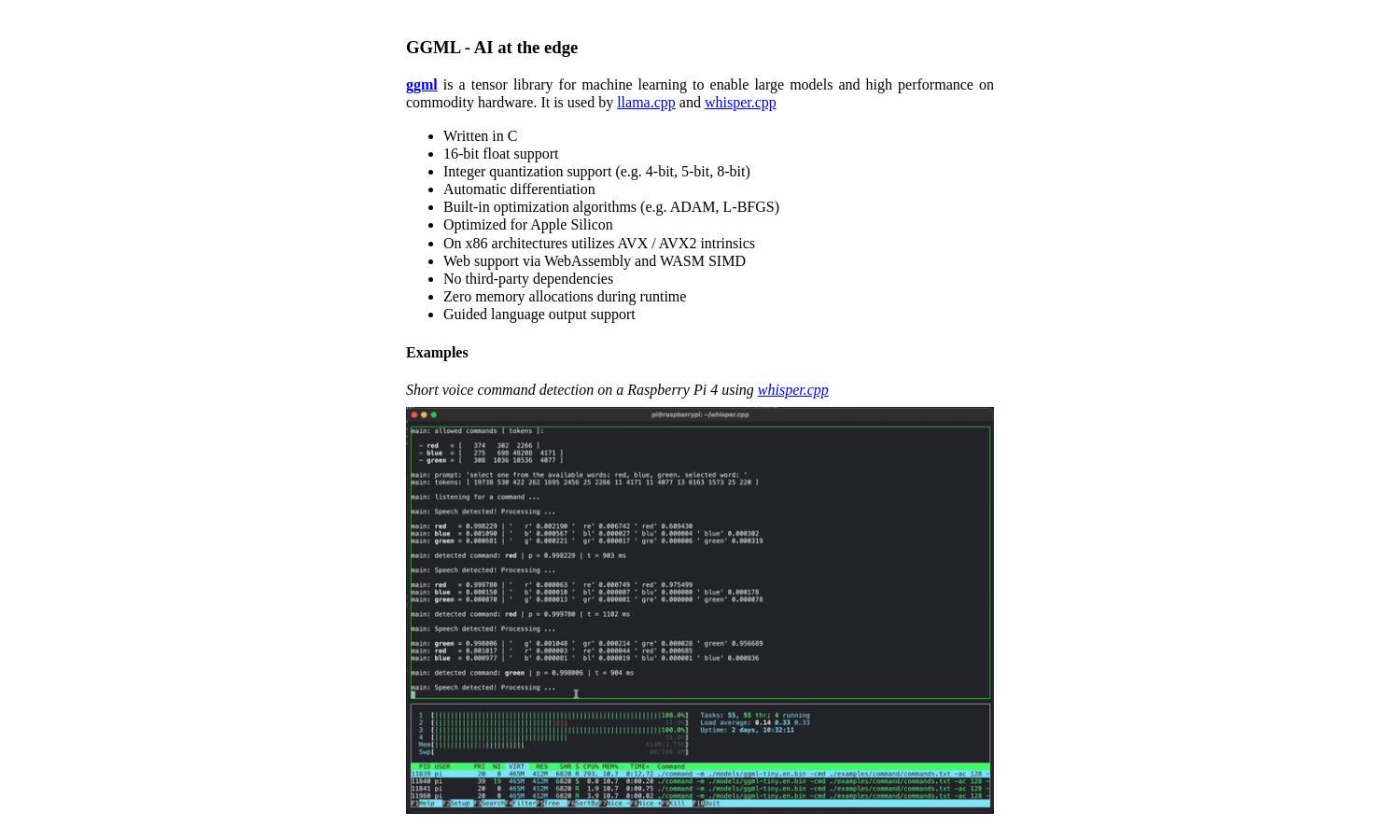
About ggml.ai
ggml.ai is an open-source tensor library designed for efficient on-device machine learning. Targeted at developers, it simplifies complex AI model execution on various hardware by ensuring high performance and minimal resource consumption. With features like integer quantization, ggml.ai stands out in enabling accessible AI.
ggml.ai offers a free and open-core model under the MIT license with potential future commercial extensions. Currently, it focuses on community contributions and user engagement without traditional pricing tiers. Users can support the project by sponsoring contributors instead of selecting a subscription plan.
The user interface of ggml.ai prioritizes simplicity and ease of navigation. With a minimalist design, it allows developers to quickly access project resources and documentation, enhancing the overall browsing experience while focusing on usability and practicality for machine learning tasks.
How ggml.ai works
Users engage with ggml.ai by first accessing its open-source tensor library, which requires no financial commitment. After onboarding, they can explore documentation and utilize its features, such as integer quantization and automatic differentiation. The library’s simplicity allows for seamless integration into existing projects for efficient on-device inference.
Key Features for ggml.ai
High Performance on Commodity Hardware
ggml.ai uniquely leverages high performance on everyday hardware, allowing developers to run large AI models efficiently. This capability empowers users to push the limits of machine learning without the need for expensive infrastructure, making advanced AI accessible.
Cross-Platform Integration
With broad cross-platform support, ggml.ai ensures that machine learning models can run seamlessly across various operating systems, including Mac, Windows, Linux, iOS, and Android. This versatility enhances user accessibility and flexibility in deploying AI solutions across different environments.
Community-Driven Development
ggml.ai thrives on community involvement, inviting contributions from users to enrich its capabilities. By fostering an open development process, it encourages innovative ideas and collaboration, enhancing the library’s value and adaptability to evolving machine learning needs.
You may also like:
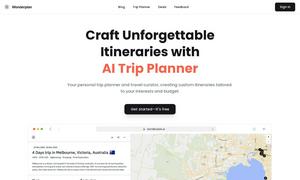