Autoblocks
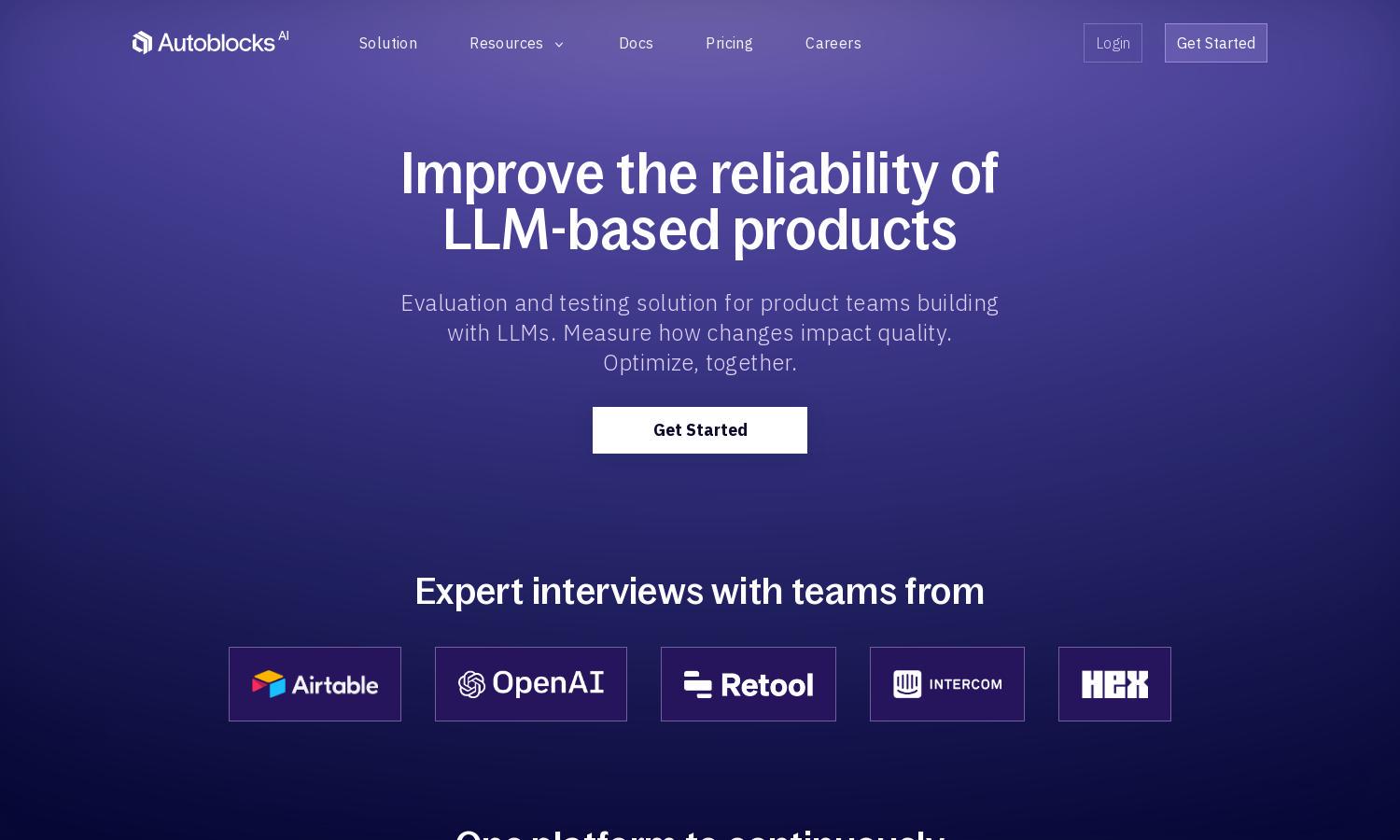
About Autoblocks
Autoblocks is a collaborative testing and evaluation platform that enhances AI product accuracy for developers. By gathering real-time feedback from experts and users, Autoblocks enables teams to create better AI solutions, streamline their workflows, and ensure their products meet user needs effectively.
Autoblocks offers tiered pricing plans catering to diverse needs. Each plan provides increasing access to advanced features, with special discounts for annual subscriptions. Upgrading benefits users with enhanced analytics, priority support, and collaborative tools that optimize AI testing and evaluation workflows.
Autoblocks' user interface is designed for seamless navigation, featuring intuitive layouts and interactive tools. Its user-friendly design facilitates effective collaboration among teams and enhances efficiency in AI product testing, making it easy for users to achieve their development goals.
How Autoblocks works
Users start with onboarding at Autoblocks, where they set up their projects and invite team members. From there, they utilize the platform's testing tools, experiment collaboratively, and gather feedback. The platform integrates with existing workflows, enabling enhancements driven by user input and expert evaluations, all while ensuring data security and accuracy.
Key Features for Autoblocks
Collaborative Testing
The collaborative testing feature of Autoblocks allows teams to gather instant feedback from users and experts. This innovative aspect enhances AI product development by aligning automated evaluation metrics with human preferences, ensuring results are accurate and relevant for end-users.
High-Quality Test Datasets
Autoblocks provides high-quality test datasets that keep pace with production realities. This feature empowers teams to identify valuable test cases and maintain observability, enhancing product quality and ensuring that development aligns with real-world user needs and expectations.
User Feedback Integration
Autoblocks uniquely integrates user feedback into the testing process, allowing for a human-in-the-loop approach. This feature aids teams in refining their AI products based on actual user experiences and expert insights, leading to enhanced accuracy and performance for LLM products.
You may also like:
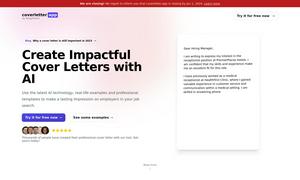